The Evolution of Fortnite From Battle Royale to Global Phenomenon
Fortnite, developed by Epic Games, started as a simple battle royale game in 2017, where...
Continue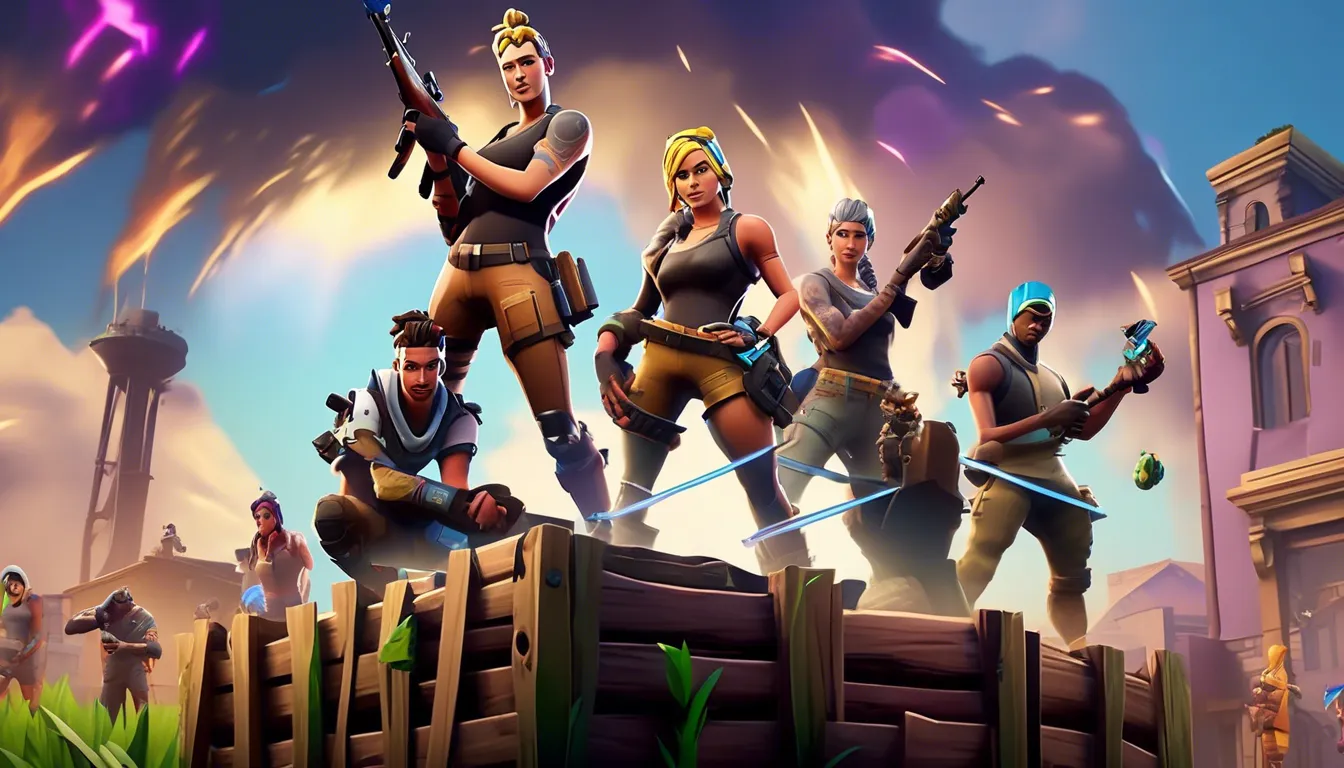
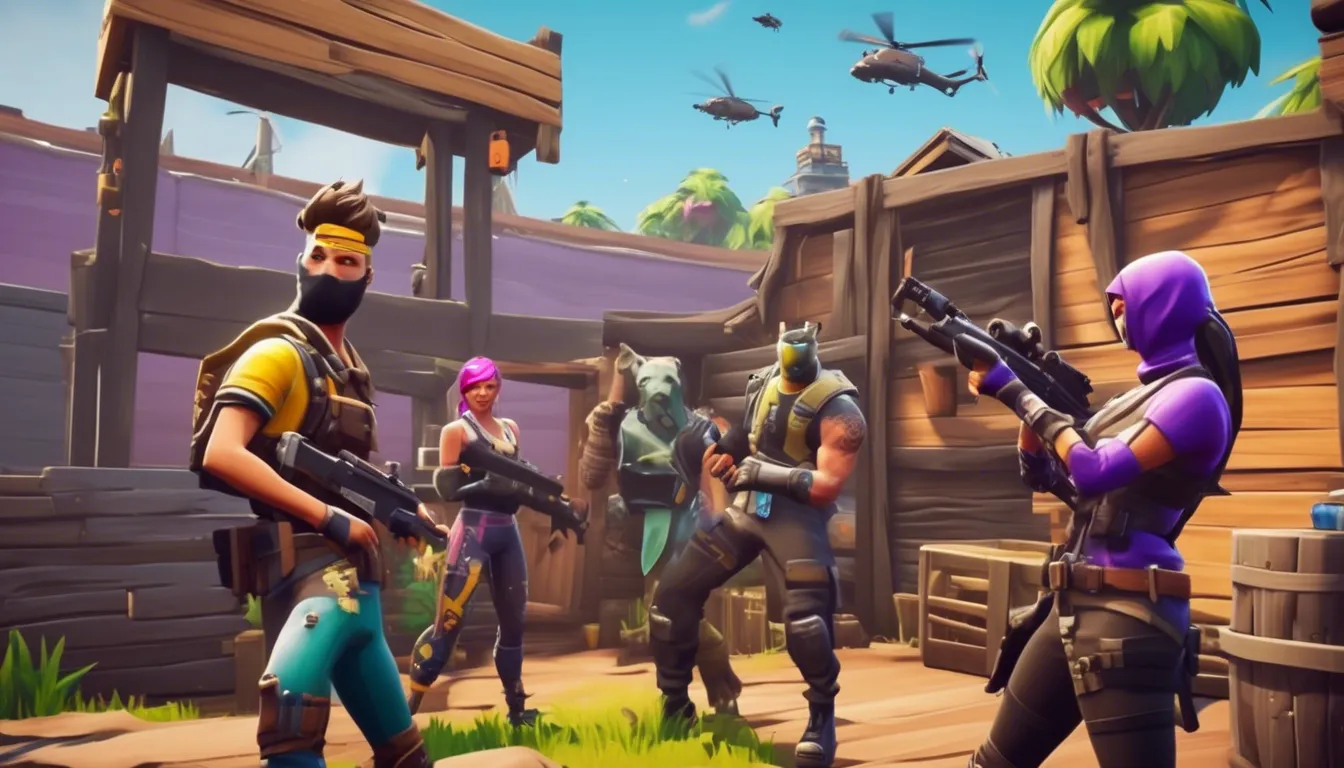
The Phenomenon of Fortnite Exploring the World of Online Gaming
Fortnite has taken the online gaming world by storm since its release in 2017. With...
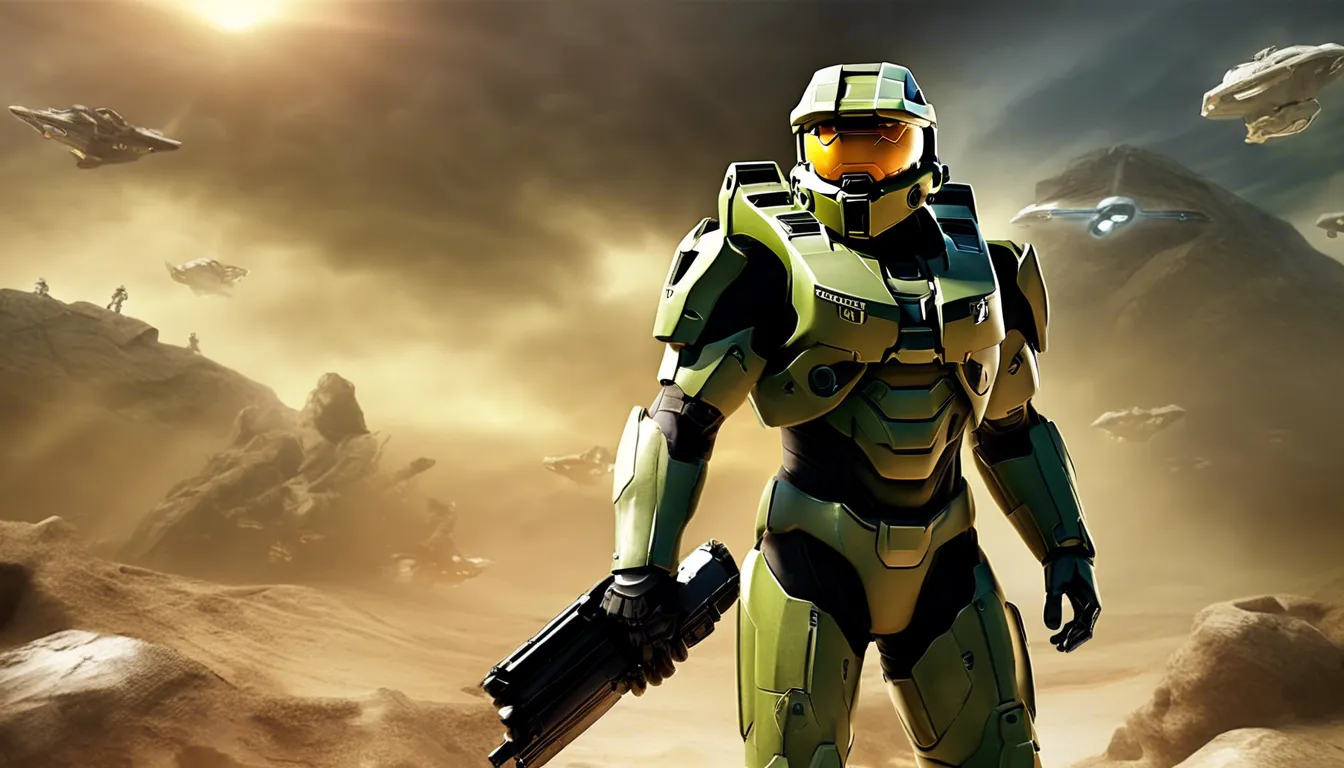
The Ultimate Gaming Experience Halo Master Chief Collection
The Ultimate Gaming Experience: Halo Master Chief Collection is a must-have for any fan of...
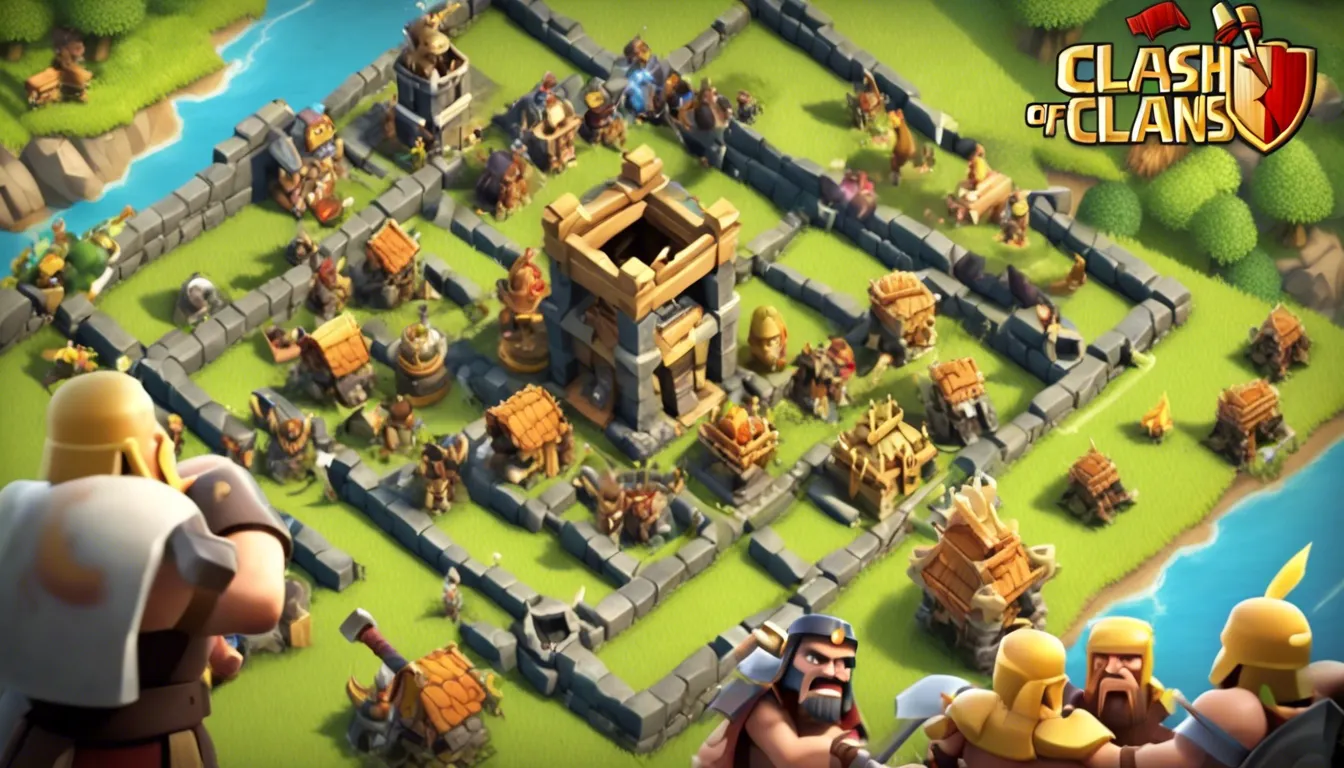
Conquer Your Enemies in Clash of Clans The Ultimate Android Game
Clash of Clans is an addictive and strategic Android game that allows players to build...
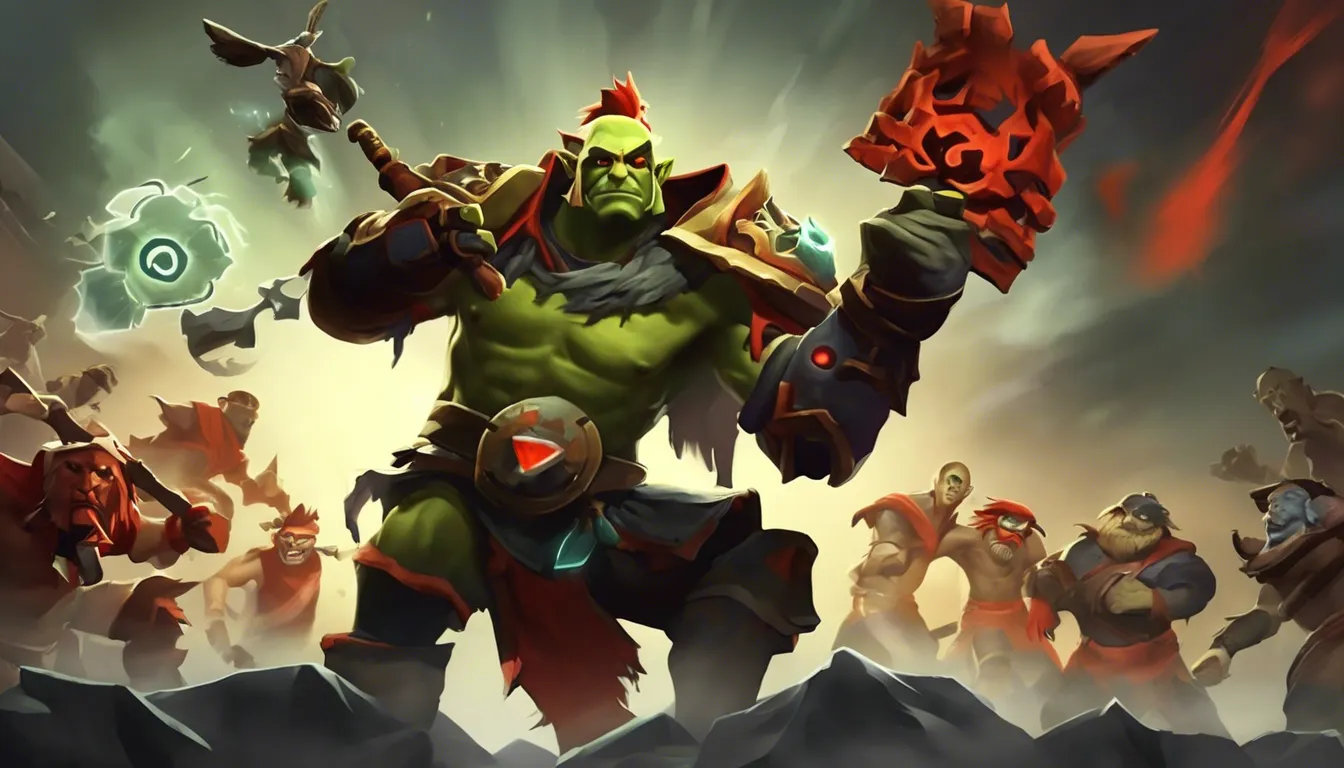
The Ultimate Guide to Dominating Dota 2 Tips and Tricks
Dota 2 is a complex and challenging game that requires strategy, skill, and teamwork to...
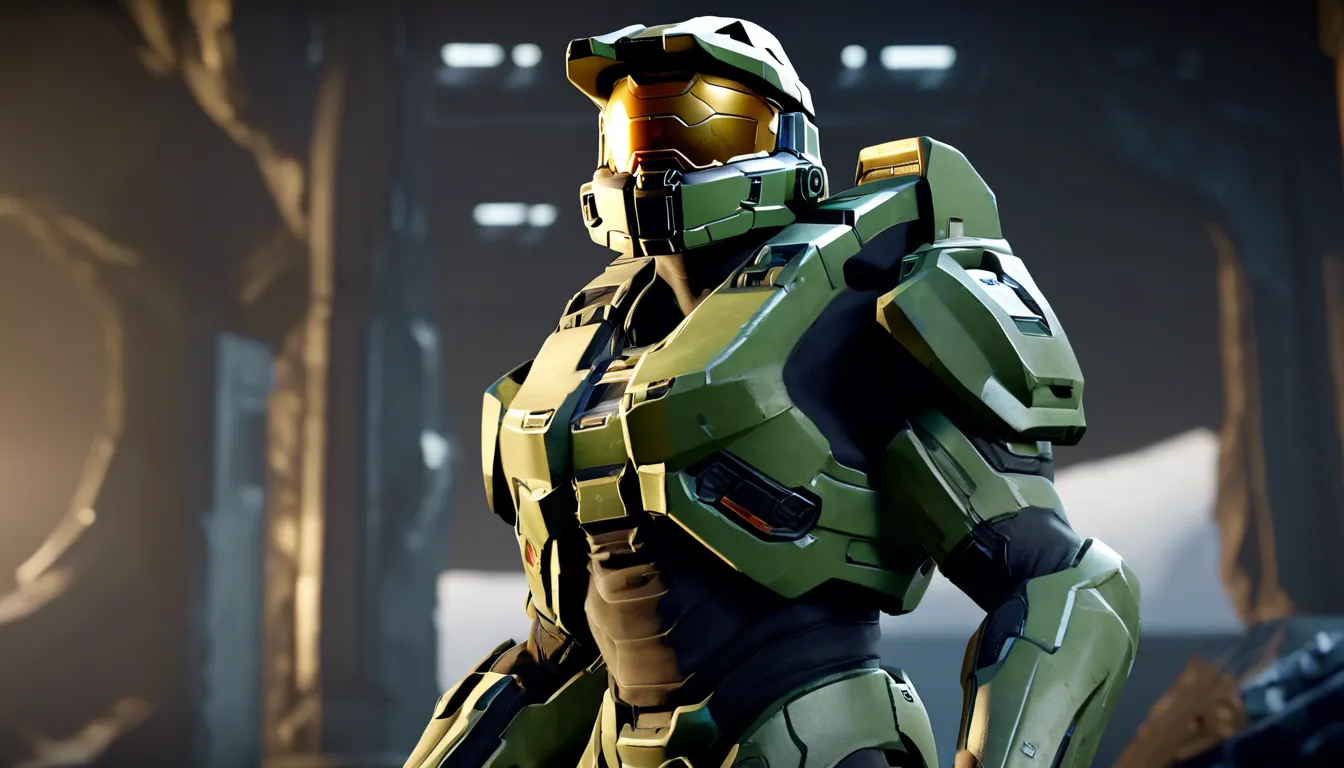
Master Chief Returns Halo Infinite Revitalizes Xbox Gaming
After a long anticipation, fans of the iconic Halo video game series can rejoice as...
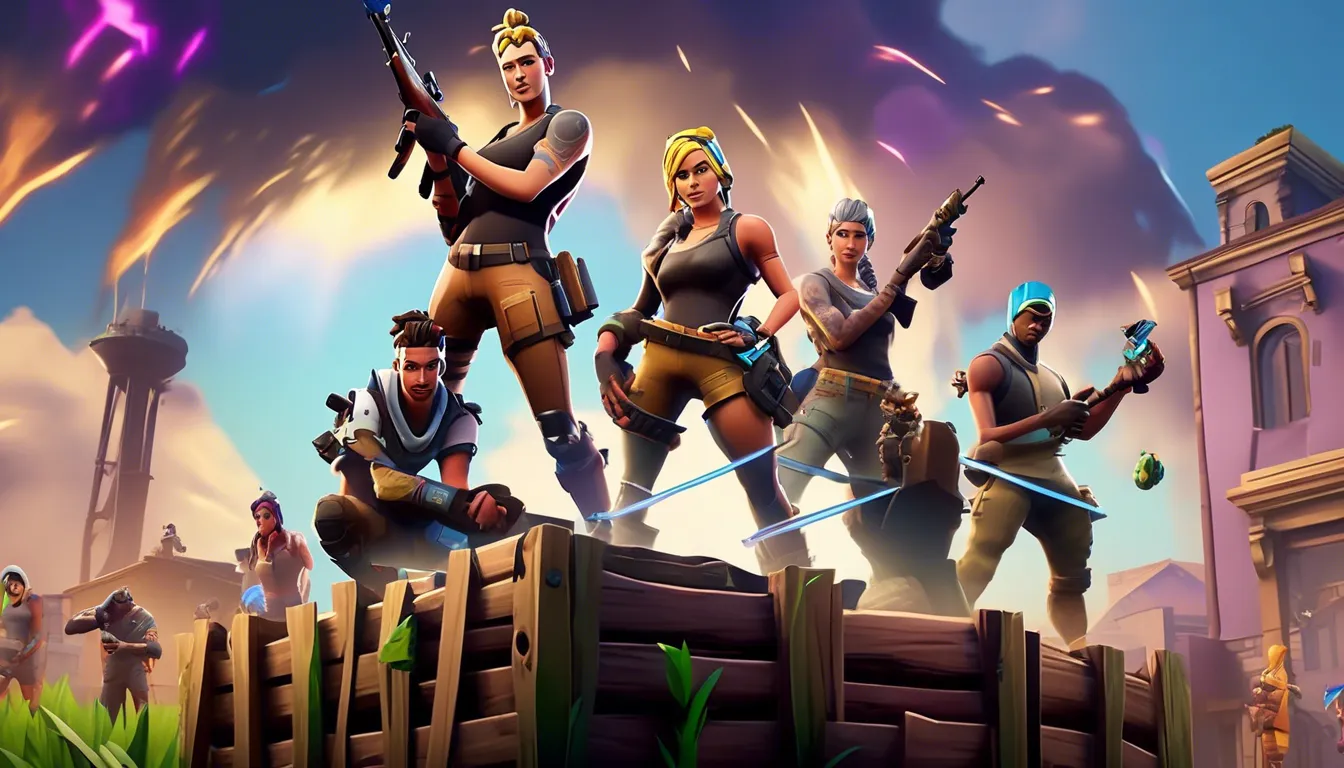
The Evolution of Fortnite From Battle Royale to Global Phenomenon
Fortnite, developed by Epic Games, started as a simple battle royale game in 2017, where...
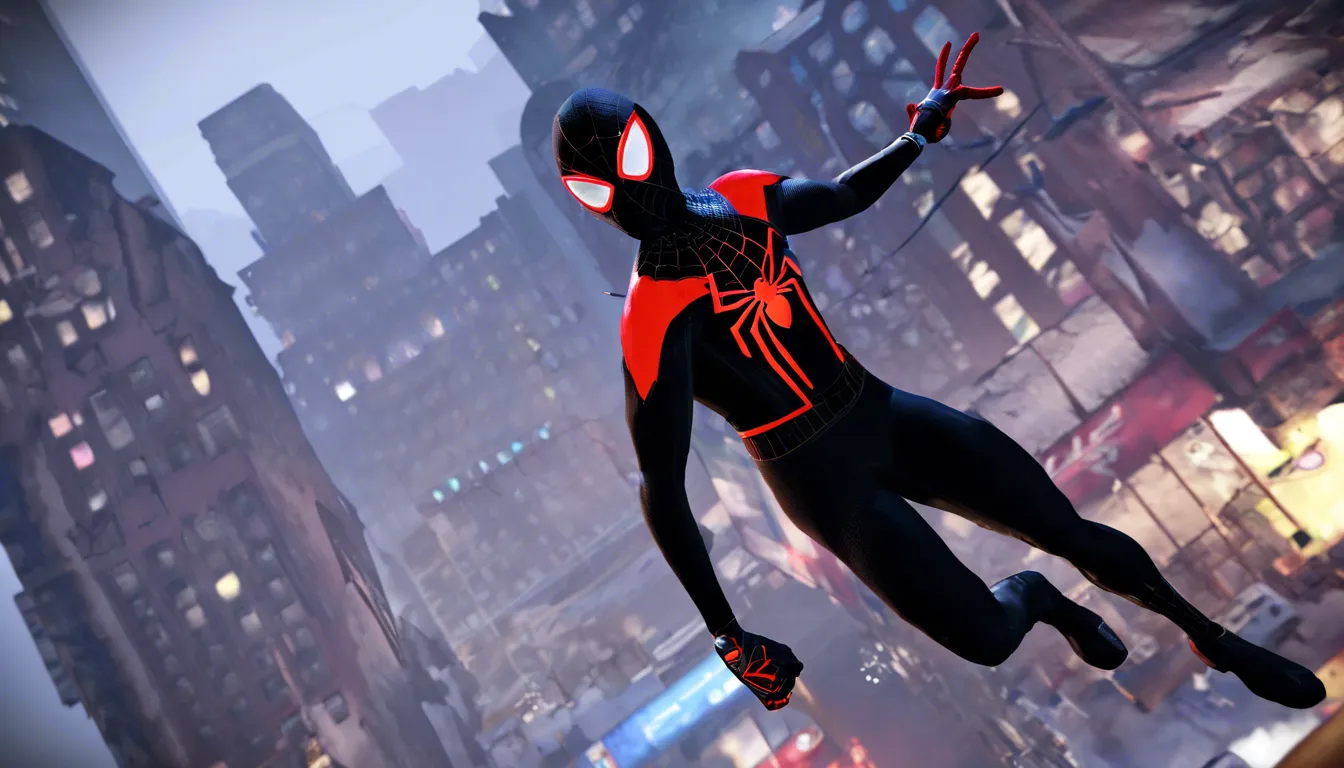
Swinging into Action A Review of Spider-Man Miles Morales
takes players on an exhilarating journey through the streets of Harlem as they step into...
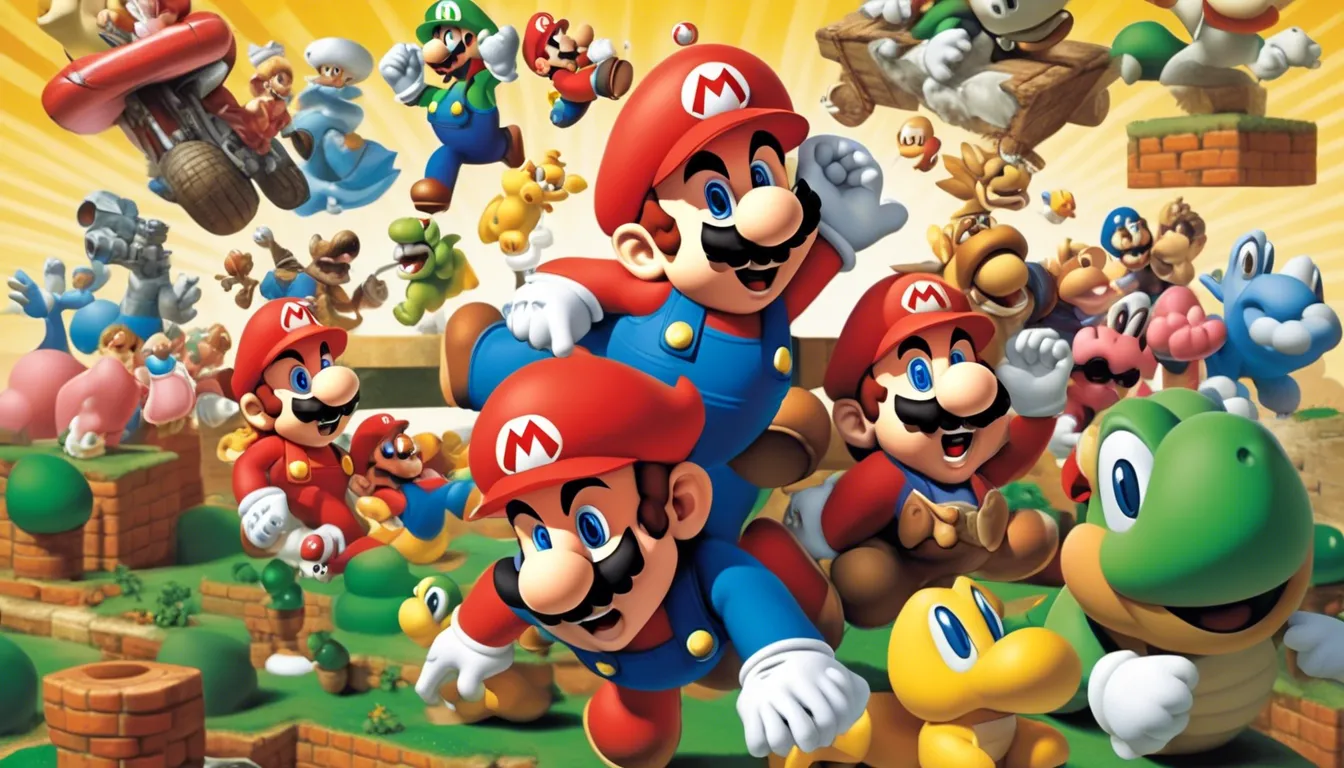
Rediscovering the Magic of Super Mario Bros.
Super Mario Bros. is a beloved classic that has captured the hearts of gamers for...
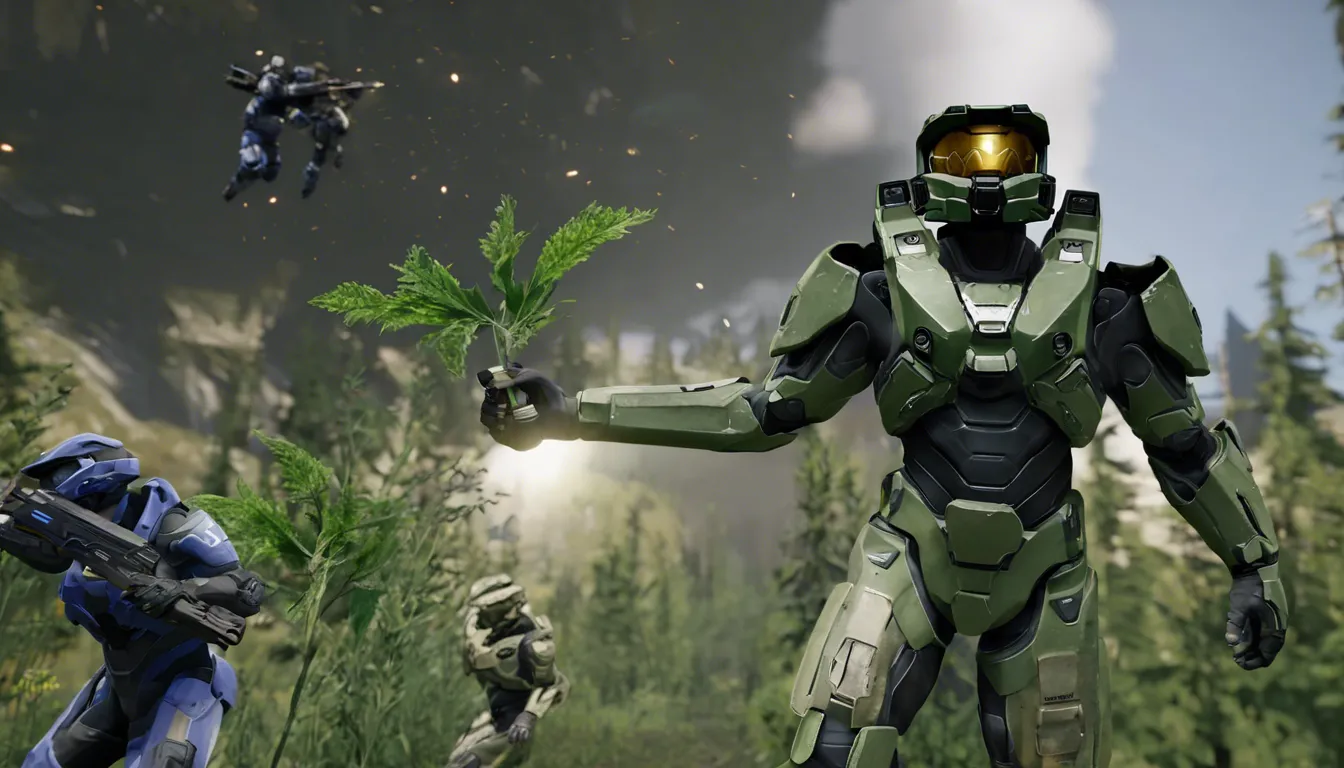
Unleashing the Future Halo Infinite Xbox Game Review
“Unleashing the Future: Halo Infinite” is the highly anticipated addition to the legendary Halo video...
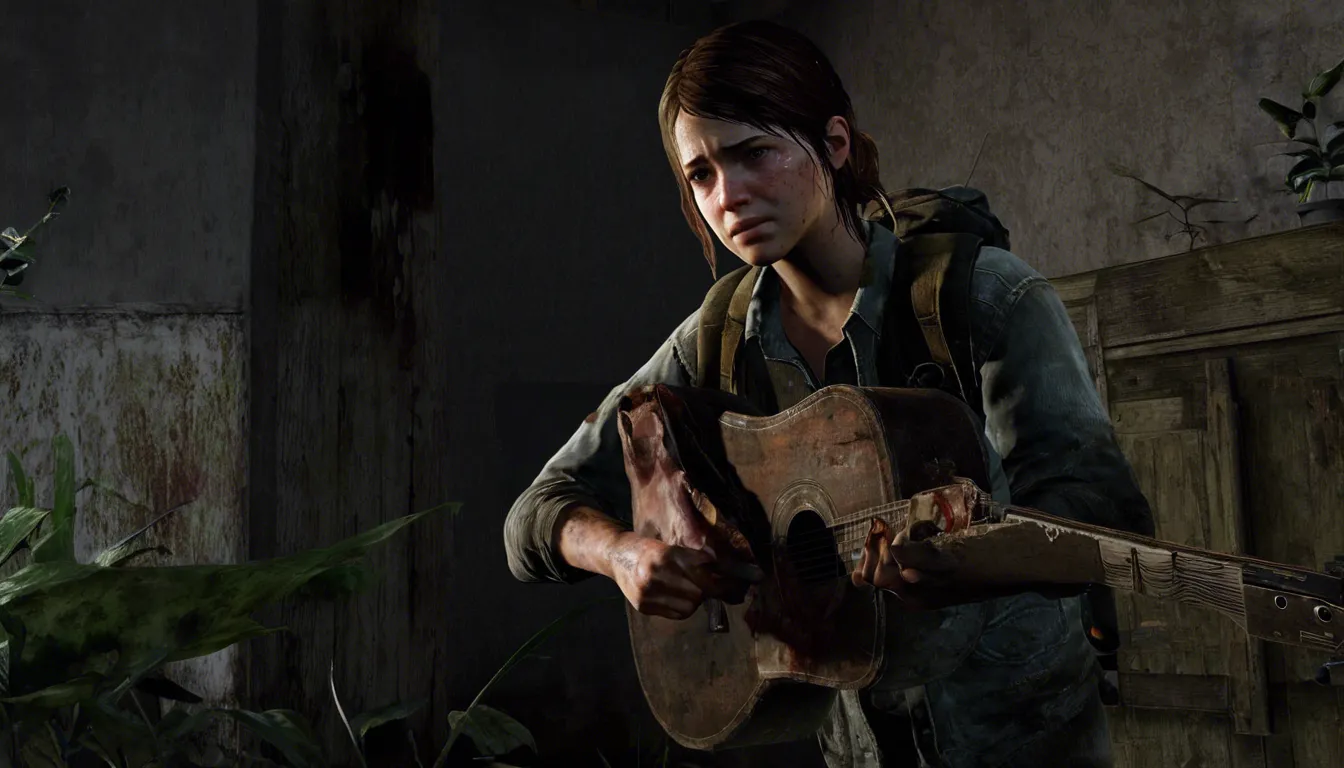
The Last of Us A Game-Changer for PlayStation Gamers
“The Last of Us” game, released in 2013 for PlayStation, has been hailed as a...